Physics-Inspired Convolutional Neural Network for Solving Full-Wave Inverse Scattering Problems
Speaker: Prof. Xudong Chen,National University of Singpore
Time and Date: 09:00 am, July 2, 2019
Place: Room 1101 of East Main Building of Guanghua Building, Handan Campus, Fudan University
Abstract:
The talk aims to solve a full-wave inverse scattering problem (ISP), which is a quantitative imaging problem, i.e., to reconstruct the permittivities of dielectric scatterers from the knowledge of measured scattering data. This talk proposes the convolution neural network (CNN) technique to solve full-wave ISPs. In order to make machine learning more powerful, a deep understanding of the corresponding forward problem is desirable. In solving ISP, the concept of induced current plays an essential role in the proposed CNN technique, which enables us to design the architecture of learning machine such that unnecessary computational effort spent in learning wave physics is minimized or avoided. Numerical simulations demonstrated that the proposed CNN scheme outperforms a brute-force application of CNN. The proposed deep learning inversion scheme is promising in providing quantitative images in real time.
Biography:
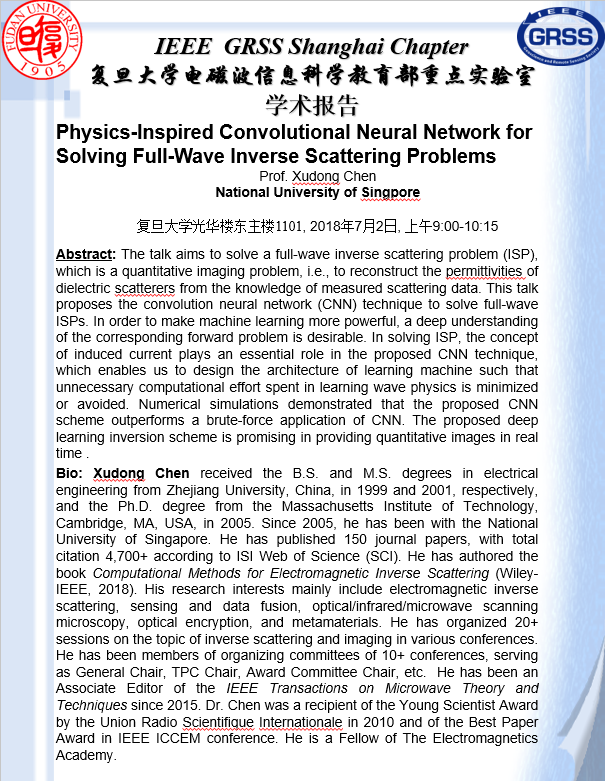